📄 First Author Paper Accepted to ISCAS 2021
Abstract
Diagnosing pre-existing heart diseases early in life is important as it helps prevent complications such as pulmonary hypertension, heart rhythm problems, blood clots, heart failure and sudden cardiac arrest. To identify such diseases, phonocardiogram (PCG) and electrocardiogram (ECG) waveforms convey important information. Therefore, effectively using these two modalities of data has the potential to improve the disease screening process. We evaluate this hypothesis on a subset of the PhysioNet Challenge 2016 Dataset which contains simultaneously acquired PCG and ECG recordings. Our novel Dual-Convolutional Neural Network based approach uses transfer learning to tackle the problem of having limited amounts of simultaneous PCG and ECG data that is publicly available, while having the potential to adapt to larger datasets. In addition, we introduce two main evaluation frameworks named record-wise and sample-wise evaluation which leads to a rich performance evaluation for the transfer learning approach. Comparisons with methods which used single or dual modality data show that our method can lead to better performance. Furthermore, our results show that individually collected ECG or PCG waveforms are able to provide transferable features which could effectively help to make use of a limited number of synchronized PCG and ECG waveforms and still achieve significant classification performance.
View on Arxiv: https://arxiv.org/abs/2102.01728
IEEE Page : https://ieeexplore.ieee.org/document/9401093
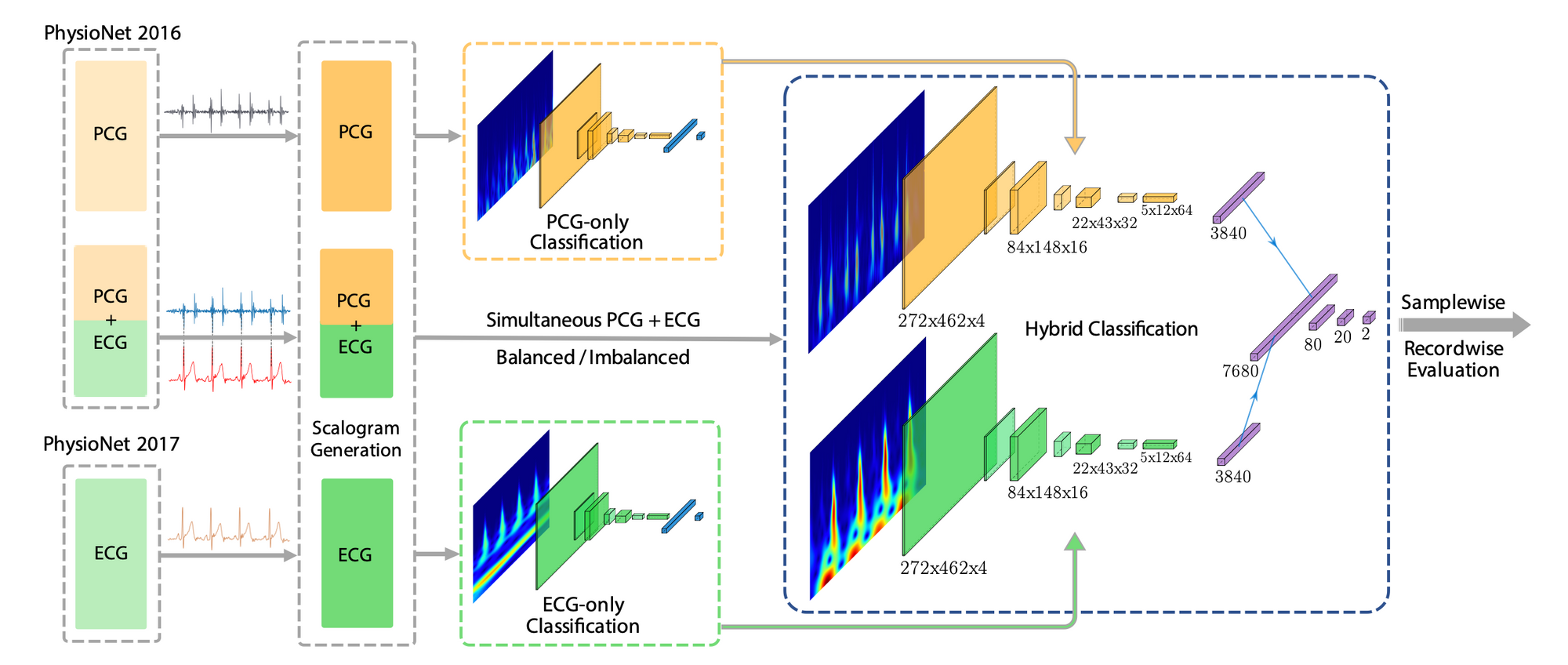