Summary of Paper - Representation Learning Via Invariant Causal Mechanisms (ReLIC)
TL;DR - Here is the the summary presentation & the annotated paper.
Recently I started reading about latest progress in self-supervised learning (SSL) 😃. I was particularly more interested towards that area due to limitations in supervised learning and the potential scalability that self-supervised learning could offer.
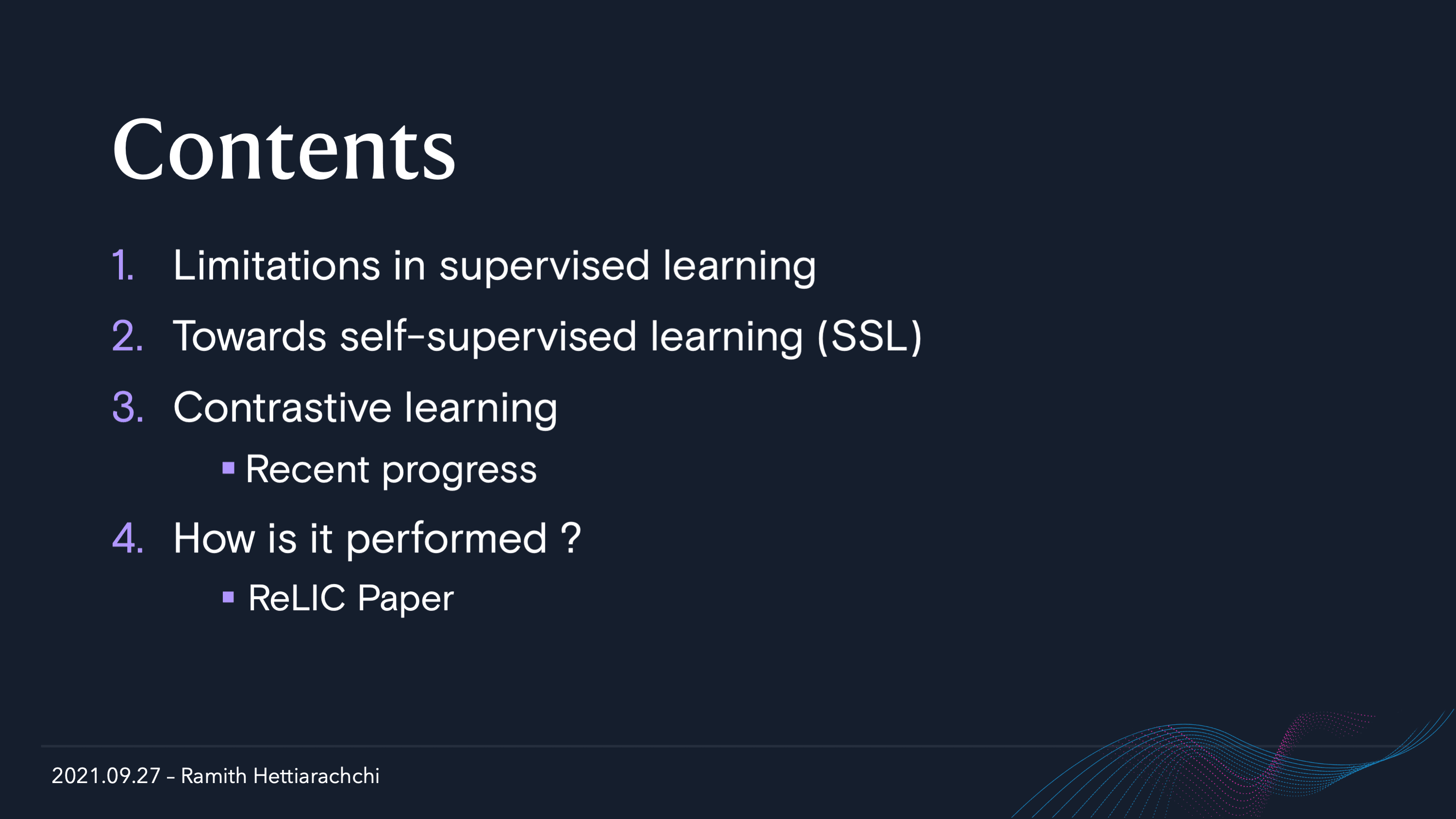
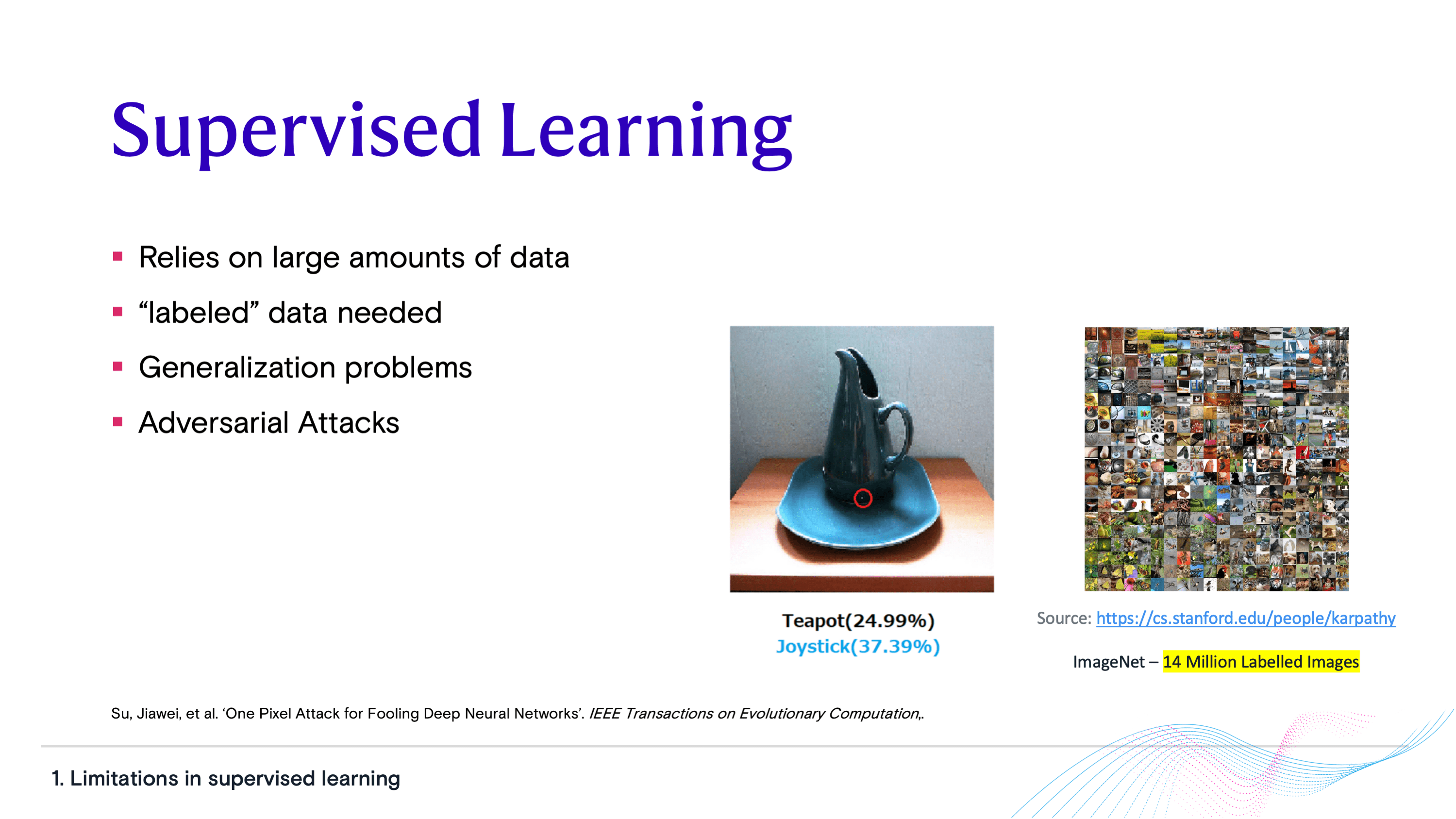
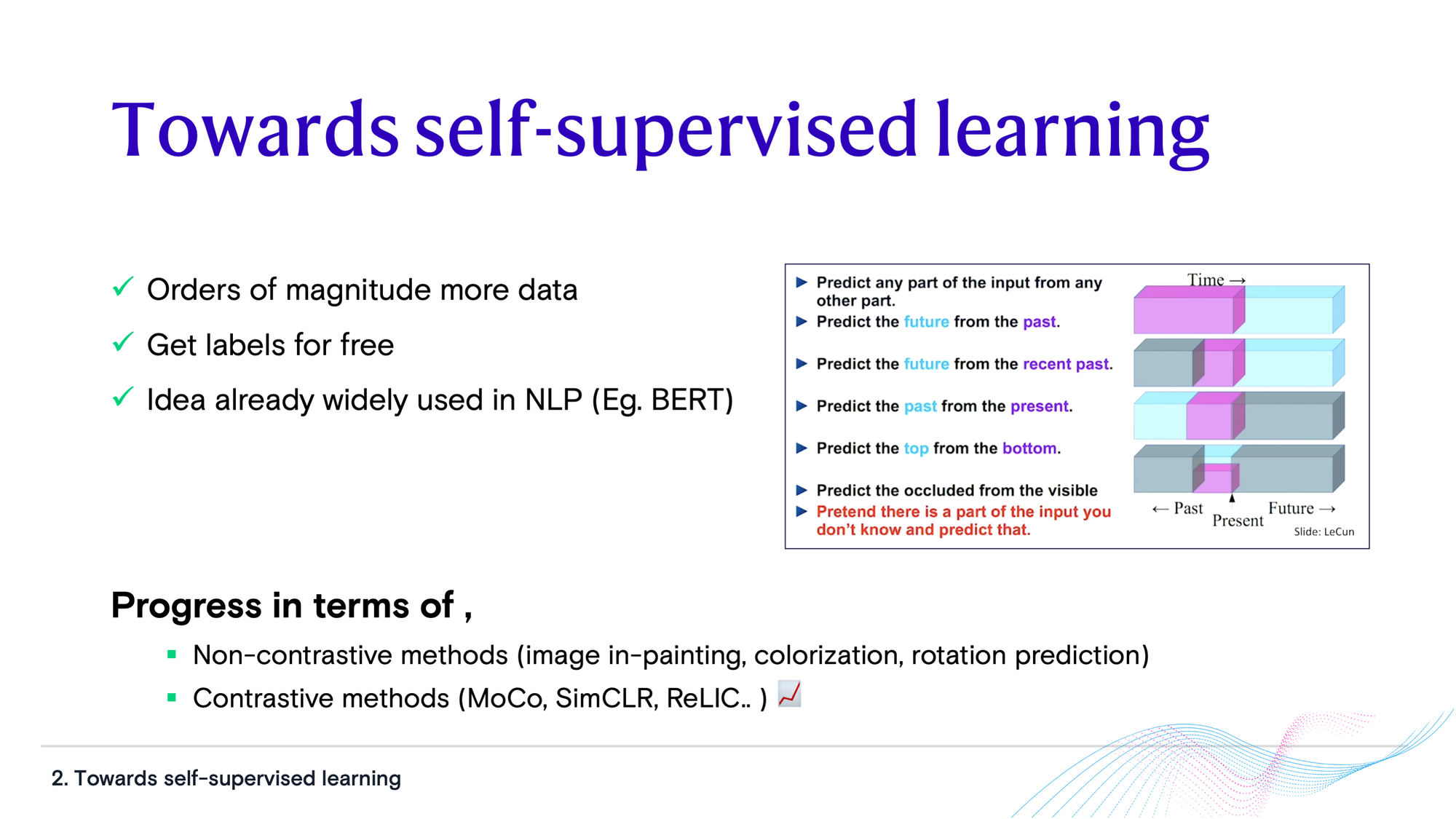
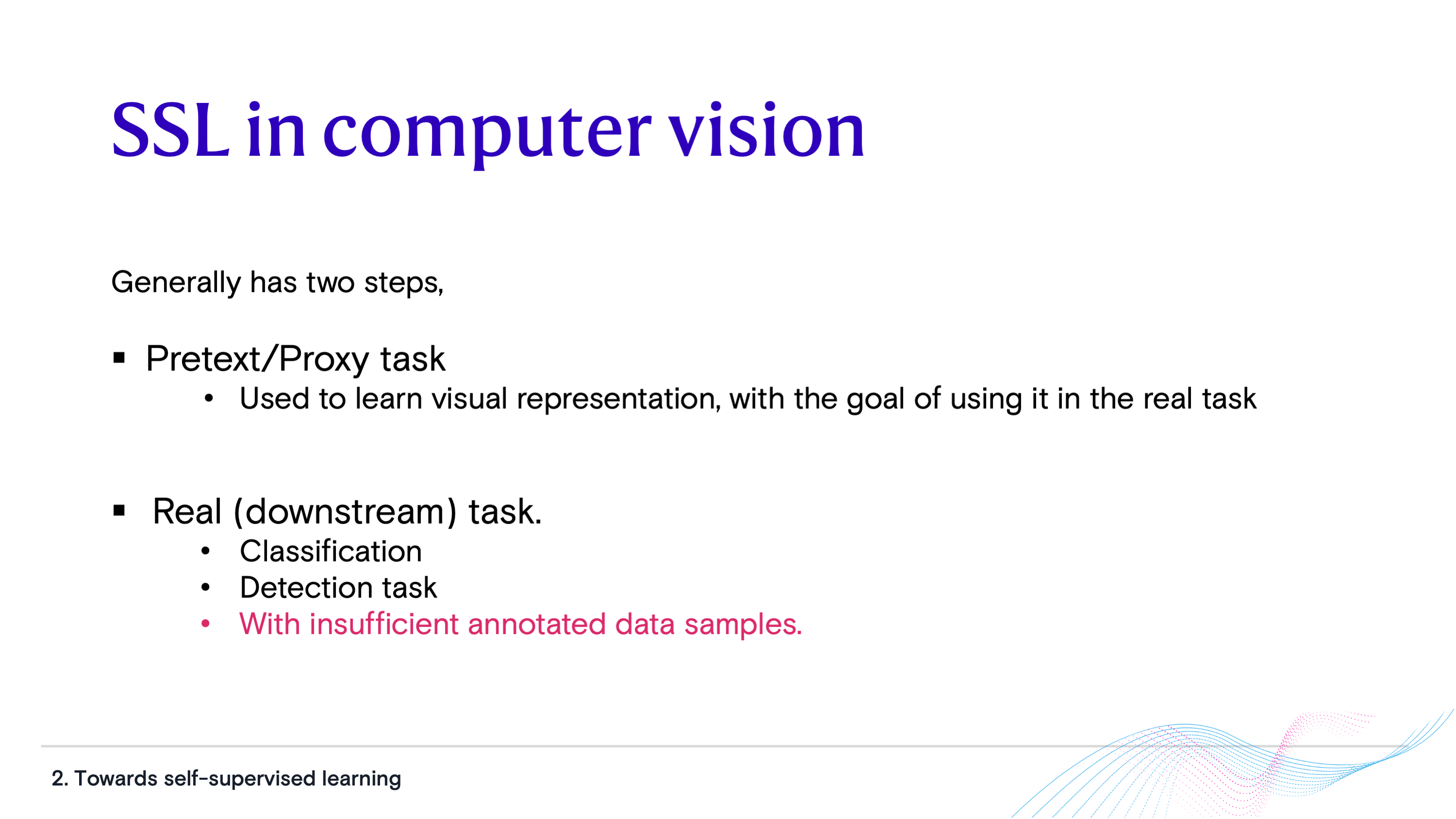
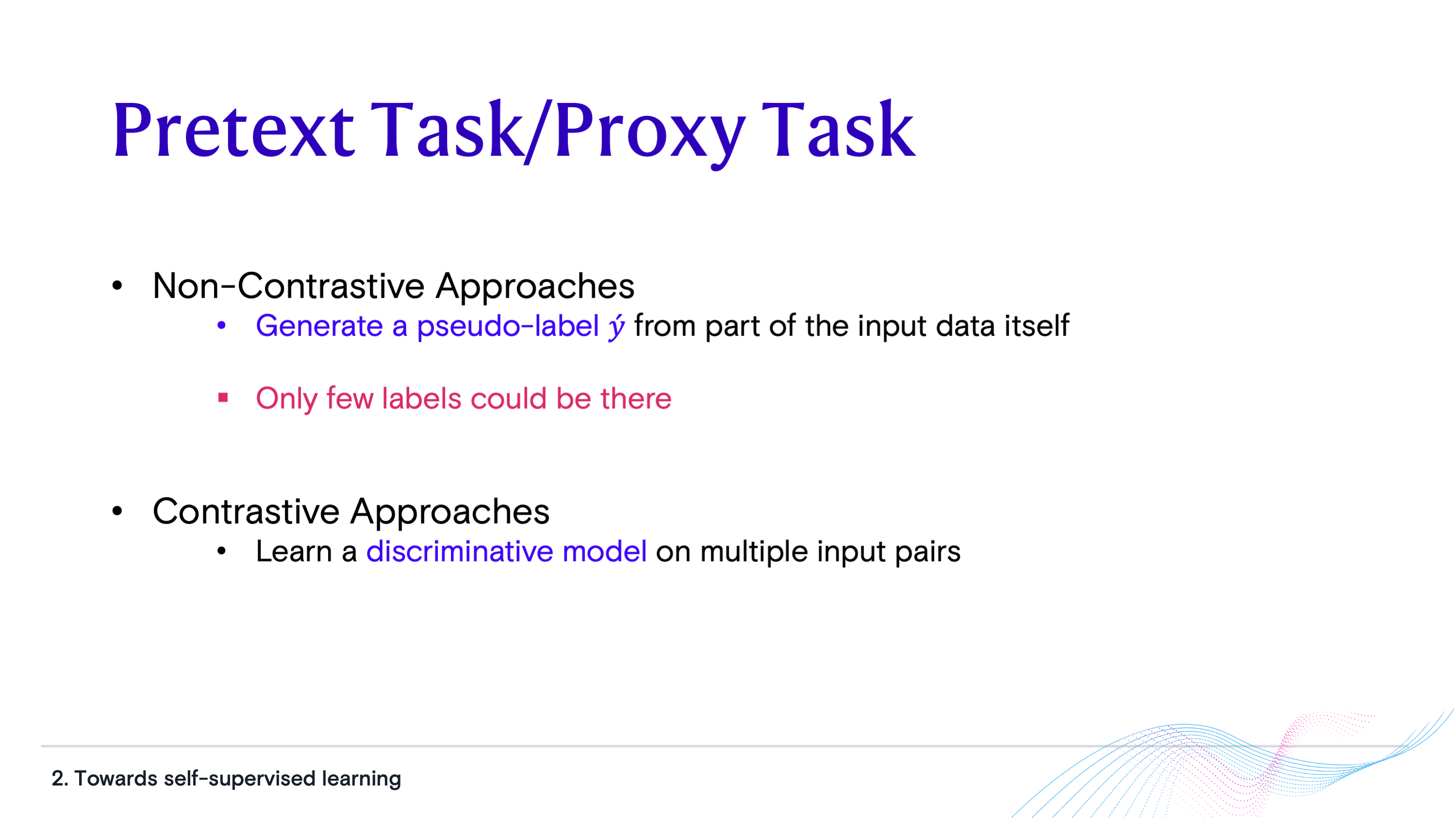
So, during the past couple of weeks I studied several papers in this area which are underlined in red below. Particularly the SimCLR [1] & ReLIC [1] papers and also a review paper on contrastive learning. Then I came up with a presentation as an introduction to self-supervised learning while summarizing the contributions of the ReLIC paper as well. I presented this during one of our weekly journal club meetings 😃. With the suggestions & feedback I got from my peers, I got motivated to write & discuss more about the papers I read.
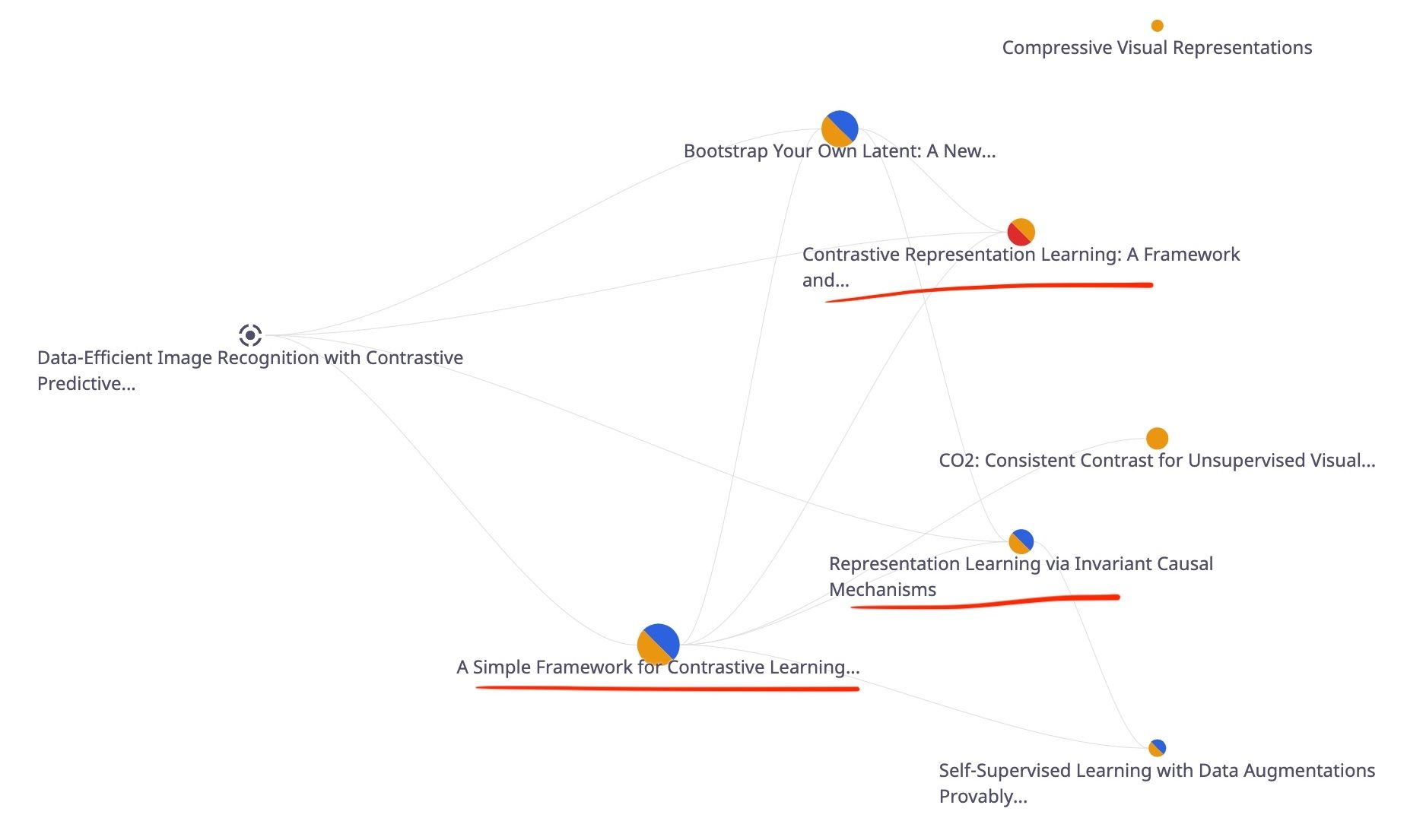
If you want to get a quick introduction to SSL and get to some details about the ReLIC paper, please check out the presentation slides below or click here to open it a new tab.